Welcome to the Machine Learning Guide for Policing
Our website is a collaborative guide for responsible development of AI in policing. We provide valuable information to law enforcement agencies, policymakers, and the general public regarding the use of machine learning in policing. Our goal is to promote transparency and accountability in the use of AI for public safety.
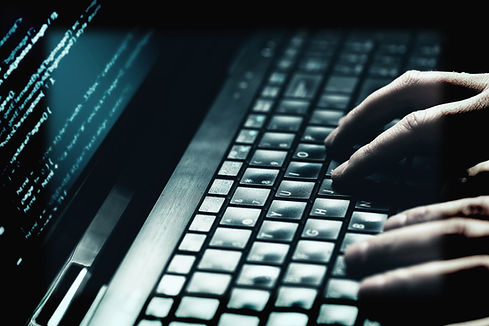
What, how, when?
There are external and internal pressures on police forces to adapt to rapid changes in technology and to adopt machine learning solutions to optimise capacity and resource issues.
​
The challenges with this range from lack of infrastructure needed for effective data science, lack of internal knowledge about what machine learning is and how it works, to lack of time to explore effective ML solutions and adopt ethical development pipelines suggested by ethical frameworks.
​
ML can be an incredibly useful tool for analysing patterns in your data for optimising staffing, discovering best practices, as well as, the traditional risk assessment and resource allocation tasks.
​
We aim to help and guide you through the analysis and upskilling required to get your force up to date with the latest requirements and techniques.
​
On this site we introduce tools for ethical development of machine learning based solutions for policing. For AI to be ethical it first has to be the right solution, at the right time, for the right reasons.
Fairness and Equity
An ethical framework ensures that algorithms are designed and implemented with a commitment to fairness and equity. Mitigating biases and striving for unbiased decision-making fosters a more just and equitable system.
Comunity Trust and Engagement
Involving community stakeholders in the development and deployment of algorithms fosters trust between law enforcement and the public.
Transparency and Accountability
Transparency fosters accountability and is vital for building trust in algorithmic decision-making processes. Encouraging the development of algorithms that are transparent and explainable allows stakeholders to understand how decisions are reached.
Mitigation of Bias
Biases in data and algorithms can lead to discriminatory outcomes. Proactive steps should be taken to identify, address, and mitigate biases in both data and algorithms. This ensures that law enforcement technologies do not disproportionately impact certain demographics or communities.
Legal Compliance
People can challenge the results of the model, adhering to legal standards and good documentation is essential for ensuring law enforcement agencies can justify and explain their decision-making process.
Continuous Improvement and Adaptation
Continuous monitoring, auditing, and impact assessments, allow for the identification of ethical concerns and the implementation of improvements over time.
Prevention of Unintended Consequences
Considering potential unintended consequences of algorithmic decision-making can help prevent negative consequences and ensures that technology is used responsibly.